Introduction
Machine learning is a field of Artificial Intelligence (AI) and computer science that imitates how humans learn using data and algorithms, progressively increasing accuracy. Algorithms receive instruction to create classifications or predictions and to reveal essential conclusions in data mining projects using statistical methodologies. Machine Learning (ML) in medical research collects and manages patient data, analyzes healthcare trends, recommends medical treatments, and more. Hospitals and healthcare organizations are starting to observe machine learning’s ability to improve decision-making and minimize risk in the medical area, resulting in many novel and intriguing career possibilities.
Although terms such as “artificial intelligence” and “machine learning” may initially seem terrifying, many machine learning principles depend on simple mathematical and programming concepts. When you have a solid understanding of the fundamentals of machine learning, you may develop the necessary skills to deal with more complex ideas and problems. This methodology may reveal novel opportunities for creativity and a range of job options in the medical field.
What makes Machine Learning more Reliable?
- Aims to enhance patient outcomes.
- Potential to unlock previously unattainable medical insights.
- Uses algorithms that predict to validate doctors’ perceptions and decisions.
- Devise a treatment plan by leveraging a patient’s medical history to identify individuals with similar conditions who have responded well to specific drugs.
In the upcoming future, ML may be able to diagnose breast cancer quicker than a biopsy. As a result of ML, paralyzed individuals could regain their independence by using prosthetics controlled by patterns found in brain scan data. In addition to these possibilities, ML research promises to help people live healthier lives. Despite the increasing number of studies on ML, its use in medical settings has remained unchanged.
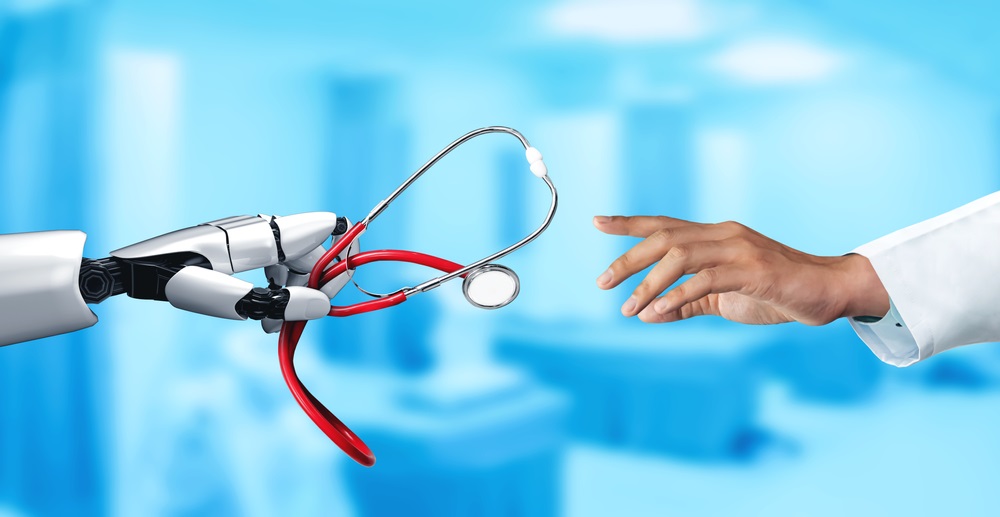
Application in Healthcare
Machine learning in healthcare focus on a fluctuating patient data set. This data can be used to identify trends that will help healthcare professionals diagnose new diseases, make risk-based decisions, and determine the effects of treatment. Because of the enormous number of patients and the broad range of medical technologies used to collect data, having medical devices synchronize to a central “network” is a convenient approach for consolidating vast volumes of data.
The (IoMT) Internet of Medical Things implies a network of medical devices and apps that can connect through the Internet. Many medical devices now have Wi-Fi, allowing them to communicate with devices on the same network or with other machines via cloud platforms. IoMT enables remote patient monitoring, tracking medical histories, and tracking data from wearable devices and other applications. The IoMT is expected to grow significantly as more wearable and internet-connected medical devices hit the market.
Applications in Medical Research
As new machine learning applications come up regularly, some of the most prevalent uses in medical research are focused on improving healthcare standards and patient health outcomes. You may choose to specialize because machine learning has many medical research applications. Understanding the various uses of machine learning in medical research (such as those mentioned below) might help you determine the area of expertise that best suits your interests and professional objectives.
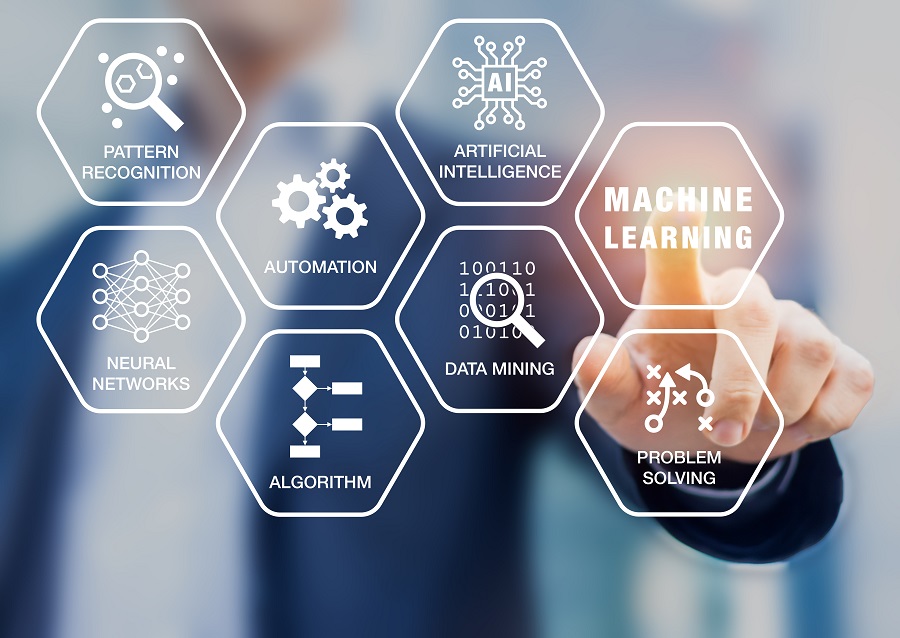
- Disease forecasting
- Biomedical data visualization
- Improving disease screening and diagnosis
- More precise health records
- Artificial intelligence-assisted surgery
- Customized treatment options for the individual
- Advancing medical research and clinical trials
- Drug development
Machine Learning Limits in Healthcare: Key Restrictions for Medical Pros
Machine learning, an algorithm that allows computers to learn from data and make predictions or judgments without being explicitly programmed, is becoming increasingly popular in AI. Machine learning, like any other technology, has limits that must be recognized before applying it in actual applications. The following are the major machine learning restrictions that every doctor, medical researcher, and healthcare expert should know:
- Human Surveillance: Although machine learning has progressed significantly in the medical field, human surveillance is still required. Surgery robots, for example, work logically rather than empathetically. Physicians and other healthcare providers may observe critical behavioural insights that aid in diagnosing or preventing medical complications.
As AI progresses, the technological and medical fields increasingly collaborate to develop the technology. Medical practitioners must have years of schooling before working in their specialities. Subject Matter Expert (SME) information complements the available data and improves explainable AI to deliver reliable and meaningful insights to the medical team.
- Ignore Social Variables: The demands of patients usually extend beyond their immediate physical circumstances. Social, economic, and historical considerations can all play a role in making suitable recommendations for specific individuals. Machine learning could assign patients to a particular treatment centre based on a diagnosis. However, this approach may not consider the patient’s financial constraints or other customized preferences.
- Data Mismatching: ML has the potential to improve any process in the medical field. Machine learning requires high-quality structured data to produce reliable predictions. Healthcare data deficits may result in inaccurate forecasts, and machine learning requires high-quality structured data. Healthcare data gaps might lead to incorrect forecasting. This has the potential to have an immense effect on clinical decision-making.
The diversity of data in healthcare is currently a barrier to mainstream ML adoption. Thousands of handwritten document scans and fragmented data could result in insufficient insights and incorrect conclusions. Furthermore, before ML algorithms can use the data, it must first be formatted and purified.
- Leads to Doctor’s Unemployment: While machine learning may help reduce expenses and alleviate clinician pressure, it may also make some positions obsolete. This variable may result in the displacement of experts who have committed time and money to healthcare education, posing challenges to equality.
Conclusion
The use of ML for diagnosis and treatment in healthcare is set to revolutionize the field. This transformative technology is already in active use, particularly for the early detection of breast, skin, and liver cancers driving higher-quality results in shorter periods.
Machine vision is increasingly becoming a crucial element in diagnostic applications. However, the applicability of this technology in the real world and the extent to which it will be used in diagnostics will be determined through trial and error.
So, Doctors, Please Share your Machine Learning Experiences in the Comments?
References
- What Is Machine Learning in Health Care? Applications and Opportunities. [Accessed on 26-07-23]. Available at: https://www.coursera.org/articles/machine-learning-in-health-care?isNewUser=true
- Pros & Cons of Artificial Intelligence [Cited on:26-07-23]. Available at: https://drexel.edu/cci/stories/artificial-intelligence-in-medicine-pros-and-cons/
- Why is machine learning trending in medical research but not in our doctor’s offices [Cited on: 26-7-23]. Available at: https://penntoday.upenn.edu/news/konrad-kording-why-machine-learning-trending-medical-research-not-our-doctors-offices