The intersection of artificial intelligence (AI) and precision medicine (PM) holds immense potential to revolutionize the healthcare industry. Precision medicine techniques discern patient phenotypes with distinct healthcare requirements or uncommon responses to treatment. (1,2) Meanwhile, artificial intelligence employs advanced computation and inference to derive insights, facilitating learning and reasoning within systems. This synergy empowers clinical decision-making through augmented intelligence. This article delves into the application and significance of AI methodologies in precision medicine.2
The Significance of AI in Precision Medicine (PM)
In clinical research and patient care, Precision Medicine (PM) is emerging as an approach that concentrates on treating and comprehending diseases by integrating multi-omics/multi-modal data from an individual.1 This integration facilitates the formulation of decisions tailored to the patient. The adoption of PM diagnostic approaches has resulted in creating of intricate and voluminous datasets, which can be effectively processed and understood through novel techniques. (1,2)
AI techniques, notably machine learning (ML), come into play to identify intricate patterns within data. These patterns can be utilized for classifications/predictions on new data or advanced exploratory data analysis.3 ML analysis of PM’s multi-modal data allows for extensive exploration of large datasets, contributing to a deeper understanding of human disease and health. (3,4)
Diagnosis and Disease Prediction
Integrating AI with PM significantly enhances disease diagnosis’s efficiency, speed, and accuracy, ultimately improving patient outcomes. (1,2) AI algorithms can process and analyze substantial medical data, including diagnostic images and patient information. They can identify subtle correlations and patterns that may escape human observation.3
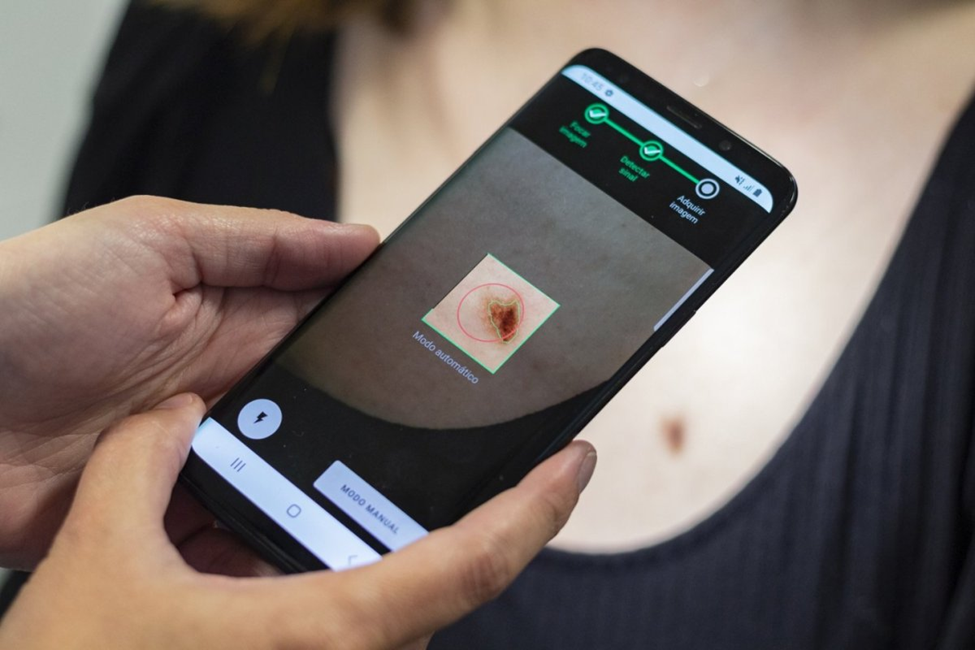
For instance, recent studies highlight that an AI model can outperform human dermatologists in accurately diagnosing skin cancer. Trained on a dataset of over 130,000 images, the AI model achieved 95% accuracy compared to the 86.6% performed by human dermatologists. This underscores AI’s potential to enhance diagnostic capabilities and offer crucial insights to healthcare professionals for timely and accurate diagnoses.1
AI is also instrumental in assessing risk factors and predicting diseases within the PM realm. By analyzing large datasets containing patient data, medical records, and genetic profiles, AI algorithms can detect patterns indicating the possibility of developing specific diseases.3,4
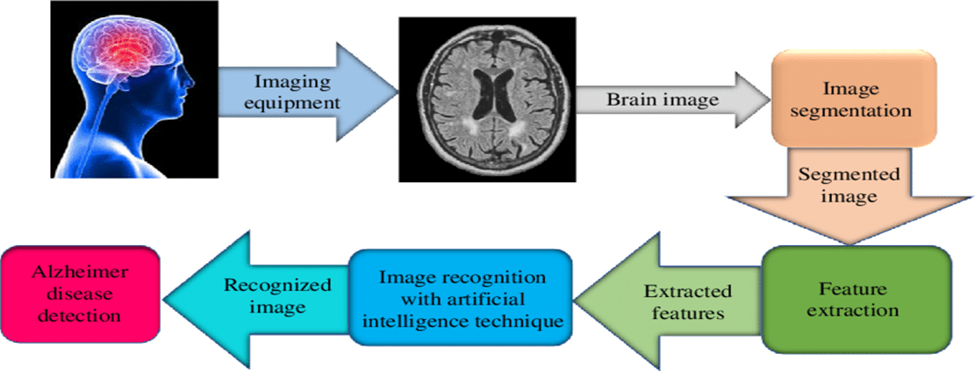
For example, an AI algorithm has been developed to predict the onset of Alzheimer’s disease several years before symptoms manifest. Analyzing patient data and brain scans, the algorithm achieved a high accuracy of 82% in identifying individuals who would develop Alzheimer’s later on. This demonstrates AI’s potential in early disease prediction, allowing for personalized preventive strategies and proactive interventions.4
Real-time Patient Health Monitoring
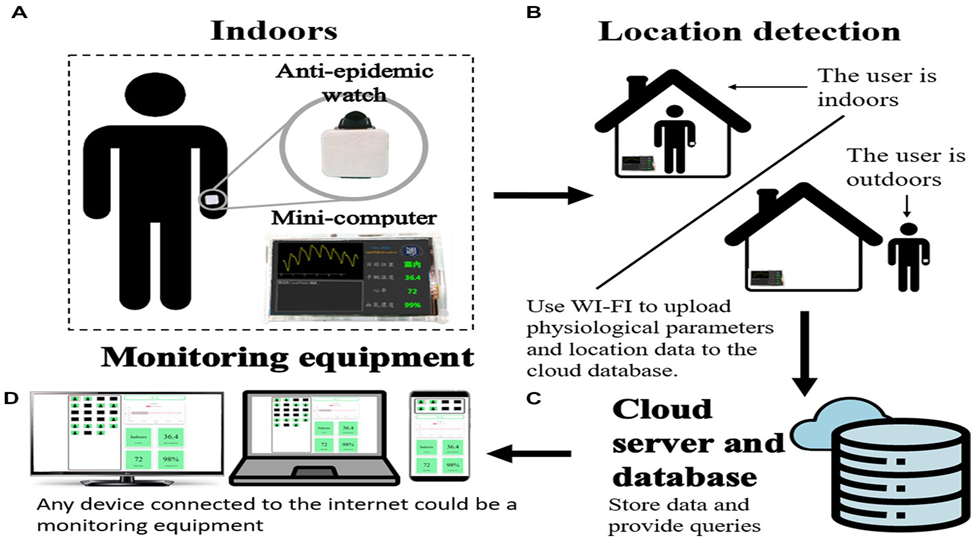
AI plays a crucial role in real-time monitoring of patient health, a key aspect of PM. AI algorithms continuously analyze incoming data streams from health monitoring platforms, wearable devices, and Internet of Things (IoT) sensors. They detect anomalies, monitor vital signs, and provide meaningful insights to healthcare professionals in real-time.5
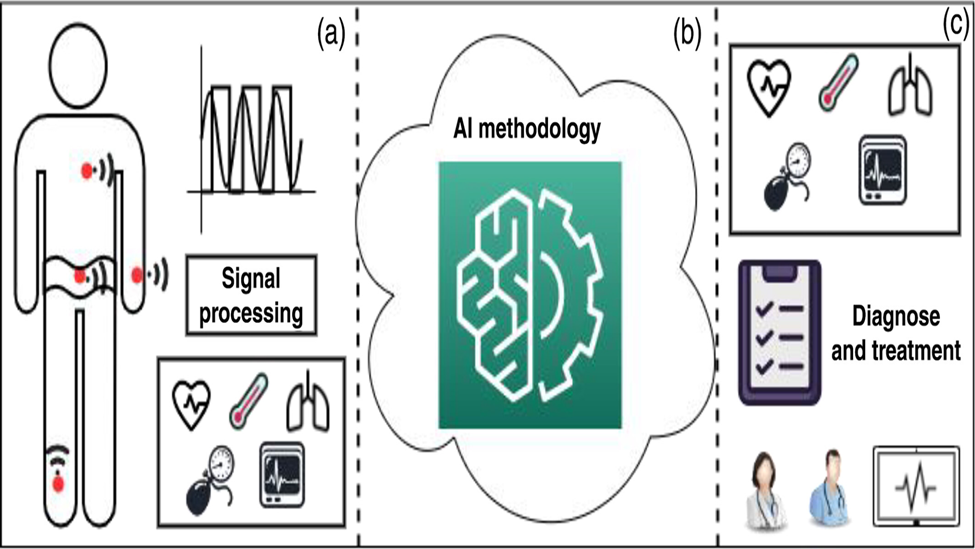
For instance, AI-driven monitoring systems can analyze physiological parameters like blood pressure and heart rate variability. This helps identify early signs of cardiac arrhythmias or abnormalities, alerting healthcare providers in real-time for immediate interventions. This approach improves patient outcomes and can save the lives of at-risk individuals.3
Furthermore, AI analyzes patient data from various sources, such as patient-reported outcomes, genomic data, and electronic health records (EHRs). This generates comprehensive insights into a patient’s health status, empowering healthcare professionals to make more informed decisions regarding personalized interventions and treatments.4
Drug Discovery and Development5
AI-powered drug discovery employs computational methods and algorithms to design, develop, and identify new drugs. By analyzing large datasets containing chemical and biological data, AI algorithms enhance the efficiency of identifying potential drug candidates.
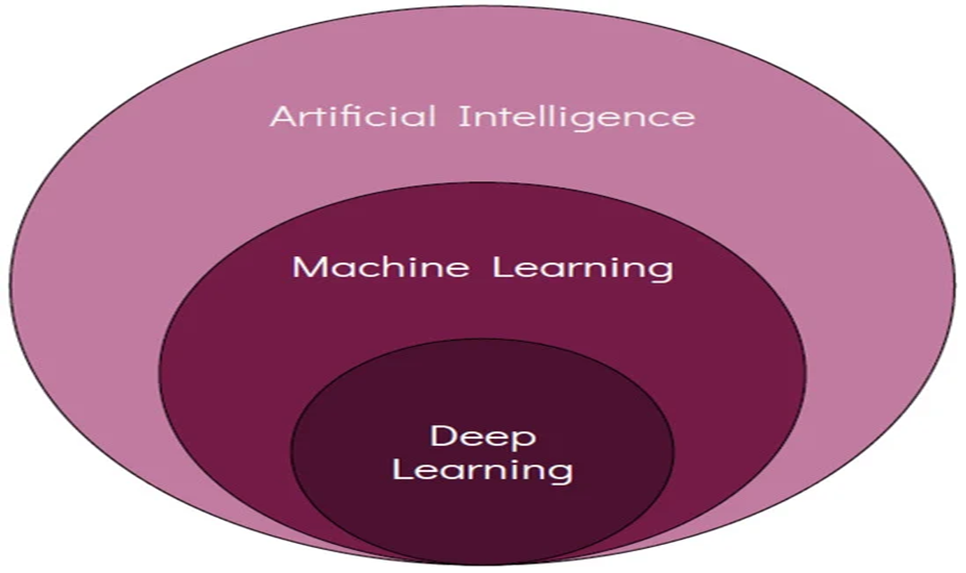
For example, different biotechnological companies utilized AI to identify a novel drug candidate for idiopathic pulmonary fibrosis. Deep learning (DL) algorithms screened millions of compounds, significantly reducing the time and resources needed for drug discovery compared to traditional methods.
AI can also identify potential drug targets, specific cellular processes, or molecules involved in disease pathways. Analyzing large-scale genomic and biological data using AI algorithms helps reveal unknown targets for therapeutic intervention.
As an illustration, a famous research Institute project employed AI to analyze molecular and genomic data from cancer patients. This led to identifying specific genetic alterations related to different cancer types uncovering new drug targets for PM.
Moreover, AI technologies optimize drug safety and efficacy by analyzing large datasets to predict drug treatment outcomes. AI algorithms personalize treatment regimens, minimizing side effects and maximizing efficacy by considering multiple factors, including treatment response data, disease characteristics, and patient genetics.
A recent study demonstrated the effective use of AI algorithms for predicting drug-induced cardiac toxicity, a major concern in drug development. The AI system predicted cardiac toxicity with over 90% accuracy by analyzing clinical data and molecular structures.
AI Techniques Employed in Precision Medicine (PM)2,4
- Support Vector Machine (SVM): SVM proves instrumental in analyzing and categorizing symptoms to enhance diagnostic accuracy. Furthermore, it finds application in identifying biomarkers for psychological and neurological diseases, validating breast cancer and multiple myeloma through the analysis of single nucleotide polymorphisms (SNPs).
SVM extends its capabilities to analyzing pathological, epidemiological, and clinical data, playing a preventive role in cervical and breast cancer. Additionally, it utilizes molecular, genomic, and clinical data for mental disease diagnosis and oral cancer validation. - Deep Learning (DL): DL is extensively used for image analysis across various healthcare sectors, particularly oncology. It is employed to scrutinize lung cancer, computed tomography (CT) scans, magnetic resonance imaging (MRI) of the pelvic and abdominal areas, mammography, brain scans, biomarker data and sequencing, glioma through histopathological scanning, and radiographs of malignant lung nodules.
DL’s applications also encompass the diagnostic process of diabetic retinopathy, histopathological anticipation in women with cytological deformations, nodular basal cell carcinoma, dermal nevus and seborrheic keratosis, cardiac muscle failure, and cardiac abnormalities. - Decision Tree (DT): DT finds its primary application in real-time healthcare monitoring, therapeutic decision support systems, and detecting aberrant sensor data. Real-time use of the DT algorithm includes therapeutic decision-making in psychological patients, identifying health outcome predictors, diagnosing hypertension through finding factors, supporting clinical decisions, locating genes associated with pressure ulcers (PUs) among elderly patients, and identifying potential telehealth services for patients.
- Random Forest (RF): RF is employed to identify nonmedical factors related to health, classify and diagnose Alzheimer’s disease, diagnose mental illness, identify factors associated with diabetic peripheral neuropathy diagnosis, predict metabolic pathways of individuals, predict mortality of intensive care unit (ICU) patients, predict disease risks from clinical error data, healthcare cost, and results of a patient’s encounter with the psychiatrist.
- Naïve Bayes (NB): NB is used for classifying Electronic Health Records (EHR), shaping clinical diagnoses for decision support, extracting genome-wide data for Alzheimer’s disease identification, modeling cardiovascular disease-related decisions, measuring the quality of healthcare services, predicting risks by identifying Mucopolysaccharidosis type II, and constructing predictive models for cancer in prostate, breast, and brain.
- Genetic Algorithm (GA): GA is applied in various medical specialties, including infectious disease, neurology, orthopedics, pediatrics, cardiology, pulmonology, surgery, gynecology, oncology, radiology, and endocrinology. Other AI techniques employed in PM encompass the hidden Markov model (HMM), linear regression, discriminant analysis, logistic regression, and k-nearest neighbor (KNN).
Recent Research Findings
A study featured in Frontiers in Artificial Intelligence showcased a population-based machine learning (ML) model designed for endometrial cancer. The research involved training seven ML algorithms using personal health data from the Prostate, Lung, Colorectal, and Ovarian Cancer Screening Trial (PLCO). The effectiveness of these algorithms was evaluated by comparing their ability to stratify endometrial cancer risk for 100 women against the performance of 15 practicing gynecologic oncologists and primary care physicians.1,3
The Random Forest (RF) model emerged as the top performer, achieving a testing area under the curve (AUC) of 0.96. It proved 2.5 times more effective at identifying women at above-average risk, with a two-fold reduction in the false-positive rate. This performance surpassed that of 15 physicians.
In a study published in Frontiers in Artificial Intelligence, researchers presented an innovative deep learning (DL) framework for planning pancreatic stereotactic body radiation therapy (SBRT). Based on 15 test cases and 85 training cases, the framework can predict a fluence map for each beam. This eliminates the need for the time-consuming inverse optimization process, resulting in an average prediction time of 7.1 seconds per patient for fluence-map prediction.1
Overcoming Challenges5
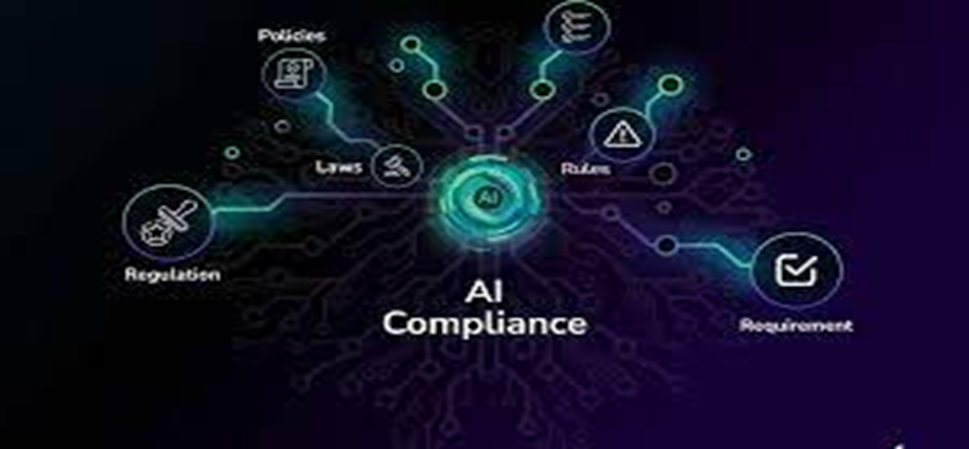
- Data Privacy and Security: Protecting patient data is a top priority. Implementing robust security measures and ensuring compliance with privacy regulations are imperative to foster trust among patients and healthcare providers.
- Regulatory and Compliance Issues: The rapidly evolving landscape of AI in healthcare necessitates clear regulatory frameworks. Ensuring adherence to regulatory standards and compliance is crucial for the responsible deployment of AI technologies.
- Transparency and Trust: Building transparency into AI algorithms is essential for gaining the trust of healthcare professionals and patients. Understanding how AI reaches its conclusions and ensuring explainaining ability enhances the credibility of these technologies in clinical settings.
Conclusion1,2,3,4,5
The integration of AI into precision medicine marks a transformative era in healthcare. By embracing the strategies discussed in this article, medical professionals can harness the potential of AI to elevate patient care. The collaborative synergy between healthcare experts and AI specialists is paramount in navigating challenges and unlocking AI’s full spectrum of possibilities in precision medicine. As we move forward, the judicious application of AI promises to revolutionize individualized patient care and redefine the landscape of medicine as a whole.
References
- How Can AI be Applied to Precision Medicine? Accessed November 14, 2023, [Internet] https://www.azoai.com/article/How-Can-AI-be-Applied-to-Precision-Medicine.aspx?utm_source=news_medical_newsletter&utm_medium=email&utm_campaign=medtech_newsletter_30_october_2023
- Hart, G. R., Yan, V., Huang, G. S., Liang, Y., Nartowt, B. J., & et al., Population-Based Screening for Endometrial Cancer: Human vs. Machine Intelligence (2020).Frontiers in Artificial Intelligence, 3, 539879. https://doi.org/10.3389/frai.2020.539879.
- Wang, W., Sheng, Y., Wang, C., Zhang, J., Li, X., & et al., (2020). Fluence Map Prediction Using Deep Learning Models – Direct Plan Generation for Pancreas Stereotactic Body Radiation Therapy. Frontiers in Artificial Intelligence. 3, 555388. https://doi.org/10.3389/frai.2020.00068.
- Quazi, S. (2022). Artificial intelligence and machine learning in precision and genomic medicine. Medical Oncology. 39, 120. https://doi.org/10.1007/s12032-022-01711-1.
- Johnson, K. B., Wei, Q., Weeraratne, D., Frisse, M. E. & et al., (2020). Precision Medicine, AI, and the Future of Personalized Health Care. Clinical and Translational Science, 14(1), 86-93. https://doi.org/10.1111/cts.12884.
About Docquity
If your educational and professional experience has not been sufficient to make your CV pop, expanding the network to other healthcare professionals to practice peer-to-peer learning might be the answer. One way to do it is by joining a social platform for healthcare professionals, such as Docquity.
Docquity is an AI-based state-of-the-art private & secure continual learning network of verified doctors, bringing you real-time knowledge from thousands of doctors worldwide. Today, Docquity has over 400,000 doctors spread across six countries in Asia. Meet experts and trusted peers across Asia where you can safely discuss clinical cases, get up-to-date insights from webinars and research journals, and earn CME/CPD credits through certified courses from Docquity Academy. All with the ease of a mobile app available on Android & iOS platforms!