Summary
Autism Spectrum Disorder (ASD) is a complex neurodevelopmental condition that affects millions of children worldwide.1,2 Early diagnosis and intervention are crucial for improving the outcomes of individuals with autism.2 In recent years, Machine Learning (ML) has emerged as a powerful tool in healthcare, making significant strides in early autism detection. This article explores how the fusion of computer vision and machine learning is transforming the landscape of autism diagnosis, offering hope for earlier interventions and improved quality of life for those on the spectrum.1,2
Introduction
In a new study published in Frontiers in Neuroscience, scientists used ML to create a novel way of identifying autism in children by studying how they choose cartoons based on their eye movements.2
It’s crucial to identify and treat ASD early, as it’s a condition characterized by difficulties in communication and repetitive behaviors. Eye tracking is an important tool for early screening and diagnosis combined with ML algorithms. Research indicates that individuals with ASD show changes in their gaze patterns when looking at socially important stimuli. This suggests exploring these patterns could lead to an early and objective diagnosis.1
Background
Traditionally, autism diagnosis relies on behavioral assessments and clinical observations, which can be time-consuming and often result in delayed diagnoses.1 Early intervention, ideally before the age of two, has been shown to improve the developmental trajectory of children with autism significantly. However, the average age of autism diagnosis worldwide is approximately four years old, highlighting the need for more efficient and accurate diagnostic tools.1,2
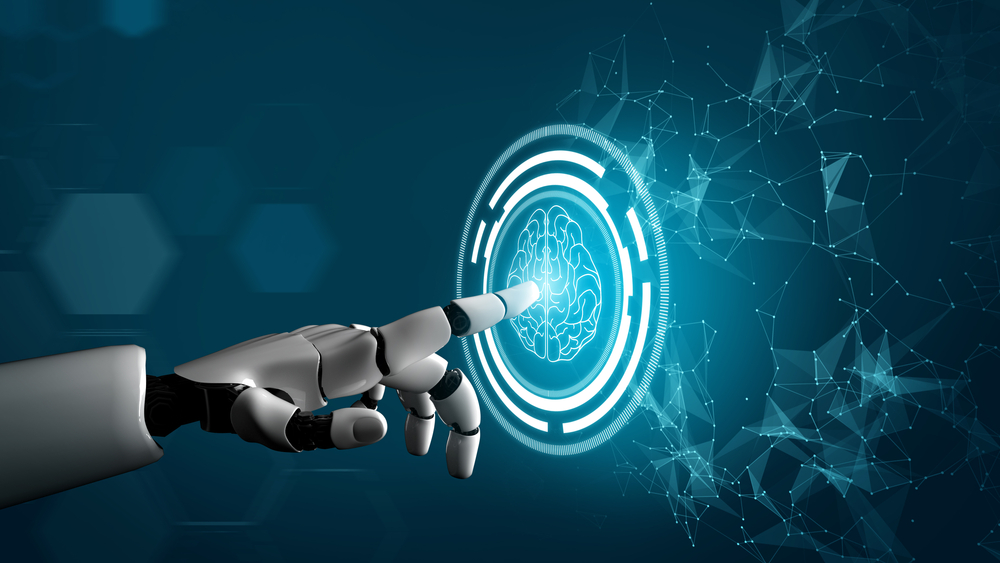
Machine Learning (ML), a subset of artificial intelligence, has the potential to revolutionize the way of diagnosing autism. By analyzing large behavioral and physiological data datasets, ML algorithms can identify patterns and correlations that may be difficult for human experts to detect. Regarding autism, ML has shown remarkable promise in leveraging one of our most powerful senses: vision.2
Research Investigation2
In this current study, as early signs of ASD include atypical gaze patterns and developmental delays, the researchers explored the potential effectiveness of utilizing minimal social stimuli, such as cartoon activities, in the early diagnosis of ASD.
The study involved assessing children with ASD and typically developing (TD) children aged between 12 and 60 months. The evaluation was carried out using eye-tracking tests featuring both cartoon characters and real individuals. The collected data from these eye-tracking assessments were analyzed using a three-tier hierarchical framework encompassing individuals, events, and areas of interest.
The researchers employed Random Forest (RF) classifiers to select relevant features, and diagnostic information and flattened vectors were used as labels and attributes, respectively. Logistic regression modeling was employed to assess the effects of the key attributes. The development of children was evaluated using the Gesell Development Scale (GDS)
The analysis included only individuals who met the criteria for an ASD diagnosis according to the Autism Diagnostic Observation Schedule (ADOS) and the Diagnostic and Statistical Manual of Mental Disorders, Fifth Edition (DSM-V). Additionally, the analysis included individuals with normal hearing and vision who could undergo eye movement evaluations. Individuals with other psychiatric conditions and significant physical health issues were excluded from the study.
Eight soundless videos were employed in our eye-tracking research. These videos were divided into two patterns, each featuring a rectangular section. On one side, a cartoon character engaged in various activities like nodding, dancing, scratching their neck, blowing a kiss, jumping, clapping their hands, bouncing while stretching their thumbs, and skipping rope. On the other side, a real person imitated the actions of the cartoon character.
To ensure that the clothing and movements in the cartoon matched those of real individuals, both the cartoon and real humans were simultaneously used in the stimulus design. “Fixation” refers to a group of gaze points concentrated in time (60 milliseconds) and space, indicating focused attention. In this context, “gaze” represents the state of not making sudden eye movements, while “fixation” signifies the same non-saccadic eye movement state.
Results1,2
Researchers recruited 161 children, including 44 typically developing children and 117 with ASD. The average age of the participants was 40 months, and 47 were female. For analysis, 70% of the data was used for training, while the remaining 30% was used for testing. Notably, the children in the ASD group were significantly younger and had considerably lower GDS than those in the TD group.
Evaluation metrics yielded the following results:
- An overall recall of 0.8
- Precision of 0.7
- Accuracy of 0.7
- An Area Under the Curve (AUC) value of 0.8
Notably, paying more attention to human-associated features was positively associated with an ASD diagnosis, whereas fixating on cartoon characters while performing certain actions, such as clapping hands, scratching their necks, counting, glancing, nodding while stretching their thumbs, and during dancing activities, was negatively correlated with ASD.
Specifically, the time participants spent fixating on people while dancing, the sequences when the cartoons nodded, and the duration of fixation on cartoon characters. At the same time, they blew kisses, indicating a favorable connection with ASD. Additionally, higher saccade velocity was associated with an ASD diagnosis.
Interestingly, children with ASD preferred cartoon faces more strongly than TD children. This preference could be attributed to their reduced need for social interaction. The shared choice for cartoon character processing in ASD may be linked to impaired social interaction abilities.
One noteworthy finding was that the duration of initial fixation on people during dancing was strongly linked to an ASD diagnosis. This association may be explained by the wider range of movements real individuals exhibit, which may appeal more to children.
Conclusions1,2
In summary, the results of this study indicate that the combination of eye-tracking methods and ML algorithms holds potential for identifying ASD in young individuals. While behaviors involving the gaze towards real humans, such as body revisits and head gaze duration, are linked to ASD, similar gaze behaviors related to cartoons, such as the sequence of cartoon fixation and the time of cartoon fixation, do not exhibit this association. These findings have social significance, as they suggest that children who show less interest in real individuals or human features may have higher chances of having ASD. Consequently, it is essential to explore computer-assisted approaches, particularly those involving cartoon characters, in the context of early diagnosis and special education for ASD.
References
- Early autism diagnosis breakthrough: machine learning uses eye movements on real and artificial faces. [Accessed on Sep 10, 2023). Available at: https://www.news-medical.net/news/20230919/Early-autism-diagnosis-breakthrough-machine-learning-uses-eye-movements-on-real-and-artificial-faces.aspx?utm_source=news_medical_newsletter&utm_medium=email&utm_campaign=clinical_and_lab_diagnostics_newsletter_25_september_2023
- Meng F, Li F, Wu S, Yang T, Xiao Z & et al. Machine learning-based early diagnosis of autism according to eye movements of real and artificial faces scanning. Frontier Neuroscience (2023). 17:1170951. doi: 10.3389/fnins.2023.1170951. https://www.frontiersin.org/articles/10.3389/fnins.2023.1170951/full